It’s time to have breakfast, but you ran out of bread. So, you quickly go to the nearest grocery, and getting through all those roads and traffic lights comes naturally. But for Artificial Intelligence, it’s not that easy. And to successfully take you to the nearest shop, a self-driving car needs to do a lot.
It has to distinguish road signs, see which light is on, watch for pedestrians on the crosswalks, and be able to follow the road markings. And it’s humans who help machines learn –– by annotating pictures and videos or labeling audio sources. In this article, we’ll find out more about the most straightforward object tagging technique –– box annotations.
What Is Bounding Box Annotation?
Millions of businesses worldwide incorporate AI-based technologies into their standard workflow. This made the global AI market in 2021 reach $327.5 billion. Just think that every Machine Learning (ML) project requires gigabytes of processed data to train computer vision.
In our example with the self-driving cars above, algorithms will require thousands of images. Those pictures have to include objects that cars will see on the way. And to make them understandable to machines, people have to mark and name them –– with bounding boxes.
In simple words, bounding box annotation is enclosing objects in rectangles (or cuboids) and naming them. According to project requirements, aA group of data annotators does this using professional software. And these requirements vary: some project owners ask labelers to put box boundaries as close as possible to an object, while others don’t mind allowing some space around it.
Customers may need to label one or several features on one object, extend its edges once hidden behind other objects (which often happens), or add depth information with 3D cuboids.
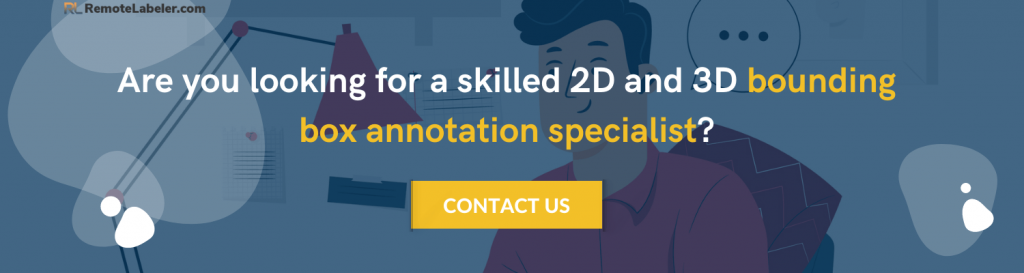
When to Use Bounding Box Labeling
Object recognition with a bounding boxes approach is prevalent because labelers can do it pretty quickly, effectively, and at scale. Moreover, this technique is the most cost-effective since it requires minimal human and computing resources.
However, not all data labeling projects are equal: some industries require deep knowledge of the topic. In this case, data annotations need to have minimal professional skills and get proper training.
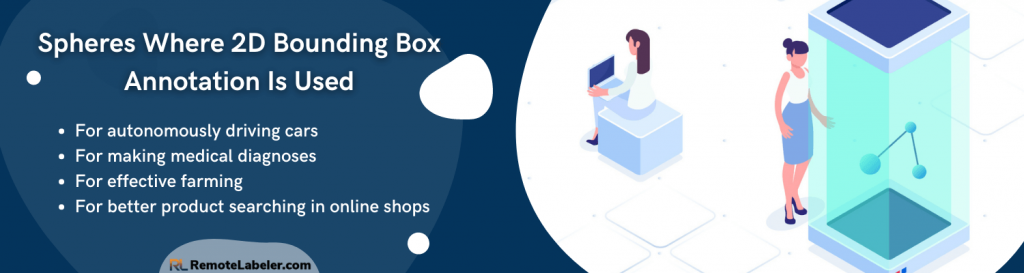
And here are the spheres where 2D bounding box annotation is used:
- For autonomously driving cars. Self-driving cars are still debatable vehicles. Though their producers recommend holding the driving wheel and remaining involved during the ride, this technology will only grow in popularity, and ML algorithms behind it will develop.
- For making medical diagnoses. Artificial Intelligence can help humans minimize medical errors because the advantage of algorithms is that they are free from any influence like emotions or tiredness. And in the context of healthcare, this means more saved lives.
- For effective farming. Drones that make pictures of large areas with seeds and crops and AI can help detect plant diseases, feed, and protect them. Moreover, farmers can select plants, adapt them to weather changes, control failures, and timely harvest.
- For better product searching in online shops. When a user searches for a jacket, bounding box machine learning algorithms offer him or her more jackets. And, the better images are tagged, the better this customer’s experience will be– and, as a result, more significant company revenue.
There definitely are other spheres of AI application, and their ML datasets are processed with bounding boxes: robotics, security video monitoring, the insurance industry, etc.
Annotation of Dataset Using Bounding Box: Pros and Challenges
Without object detection, no computer vision model can make 2D and 3D bounding box annotation a primary way to process data for machine algorithms. But aside from obvious advantages, this method has several challenges –– let’s take a look at both.
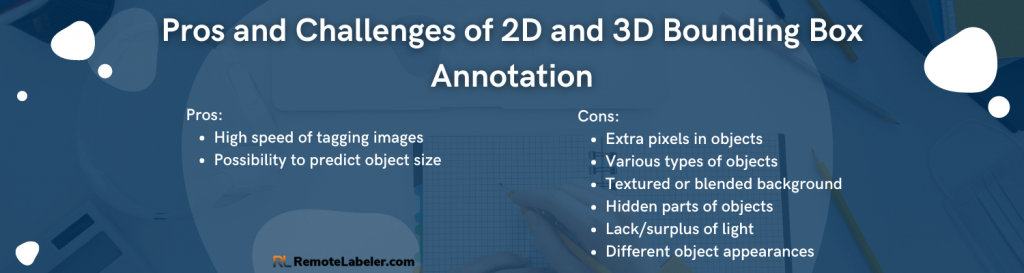
Advantages:
- High speed of tagging images. Labelers can do object localization quickly, provided that they have professional software. Still, advanced tools are more expensive.
- Possibility to predict object size. Annotators can extrapolate actual sizes of objects when their parts are hidden behind other objects.
Challenges:
- Extra pixels in objects. Rectangles with detected objects can include other objects or free space in the background, making some datasets inaccurate. When object shapes are non-linear, it’s more efficient to use polygonal or landmark annotation.
- Various types of objects. Even if it’s the same car, there are big, small, red, black, high, low, long, short, and other types of cars. And to teach algorithms properly, the dataset has to include them all.
- Textured or blended background. Overshadowed or out-of-focus objects can make the identification area inaccurate or require more time to be correctly localized.
- Hidden parts of objects. Datasets have to contain images with full-size objects and their parts. The training set has to be unbiased to teach algorithms to detect objects effectively.
- Lack/surplus of light. Poor or excessive Illumination makes object margins vague. This may cause a confusion in the identification of separate objects.
- Different object appearances. You need to add images with objects from various perspectives to teach an ML model to identify them properly.
This way, the object detection bounding box method faces several challenges. The major of them is finding the balance between accuracy and speed of tagging data.
How to Use Bounding Box in Image Processing
After images are loaded into the data labeling tool, an annotator starts identifying objects and tagging them one by one. Detectors have one or several criteria to be labeled to the image, and some platforms allow automatic tagging too. First, a user marks the upper left top of the rectangle.
With extended X and Y axes, he finds the exact spot so that the perpendicular lines embrace the object. Denoting the lower right vertex of the box is even more straightforward, and the next step is attaining labels. For example, class: a car; color: red; make: Toyota; model: Camry, etc.
Why Choose Us among Other Bounding Box Annotation Vendors
To launch a top-level computer vision project, you’ll need a professional image recognition machine learning face bounding box team. And we can help you assemble it within 2-4 weeks. We boast access to the talent pool of top data annotators from Ukraine, which offers you certain benefits.:
- You can save on payroll expenses. Since we work with Ukraine-based specialists, you can hire European specialists for competitive prices. By hiring annotators with us, you can save up to 15% of the costs related to annotating your images.
- You pay only a fixed fee. Our services embrace the administrative burden of keeping an in-house labeling team. So, you won’t have to pay salaries, report on taxes, transfer rentals, settle utility bills or arrange a working place. Instead, we offer a broken-down fixed fee.
- You pay for what you get. If you hire three annotators today and need three more tomorrow, we’re here to source them. Or, if you need to pause a project for a while, no fees are applied –– and you pay for the people you work with.
Learn how to hire a high-quality bounding box annotation team for your AI project!
- Emerging Trends and Future Outlook: The Data Labeling Industry in 2024-2030 - December 8, 2023
- Landmark Annotation: Key Points - November 6, 2023
- All You Should Know About Bounding Box Annotation - November 5, 2023