In today’s digital age, as businesses increasingly outsource data annotation services, ensuring the sanctity and security of data has become paramount. Especially in the realm of panoptic segmentation, where vast amounts of intricate data are processed, the stakes are high. Recent studies indicate that data breaches have surged by 67% over the past five years, emphasizing the critical need for robust data security measures.
The Importance of Data Security in Panoptic Segmentation
Panoptic segmentation, a cutting-edge technique in computer vision, amalgamates both semantic and instance segmentation to provide a comprehensive understanding of an image. This method offers a holistic view by classifying every pixel in an image, making it invaluable for applications ranging from autonomous driving to medical imaging. Given the depth and detail of the information processed, it’s evident that the security of panoptic segmentation datasets is of utmost importance.
Data security in panoptic segmentation isn’t just about protecting business interests; it’s also about safeguarding the privacy of individuals. With the rise of video panoptic segmentation, for instance, there’s an increased risk of exposing personal information, especially when processing footage from public spaces or private settings.
Types of Data Used in Panoptic Segmentation Projects
Understanding the types of data used in panoptic segmentation projects is pivotal to appreciate the gravity of data security in this domain. Each data type, with its unique attributes and sources, presents distinct challenges and vulnerabilities.
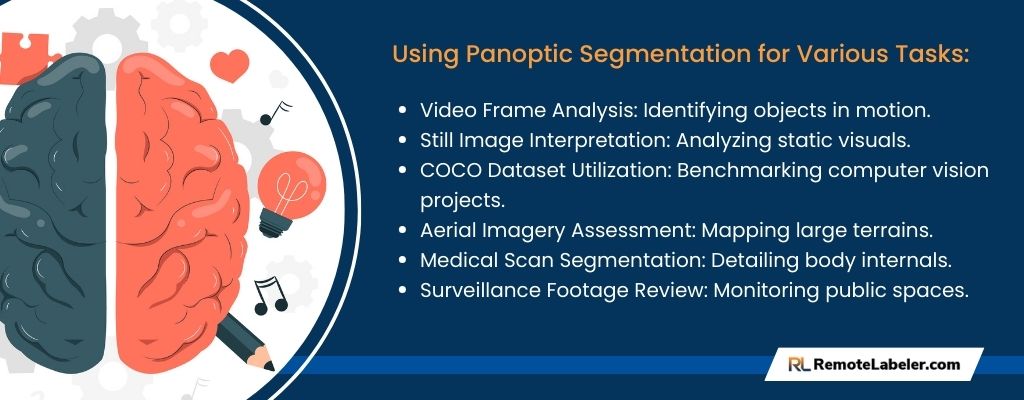
Video Panoptic Segmentation Data
Video panoptic segmentation involves analyzing video frames to identify and classify every object and its context. This data can be particularly sensitive, especially when sourced from surveillance systems, traffic cameras, or personal recordings. A breach here could compromise the privacy of countless individuals.
Panoptic Image Segmentation Data
Unlike its video counterpart, panoptic image segmentation focuses on still images. These could range from satellite images to medical scans. Given the diverse sources of such images, the data can often contain confidential information, be it about a location’s topography or an individual’s health condition.
COCO Panoptic Segmentation Data
The COCO (Common Objects in Context) dataset is a benchmark in the world of computer vision. COCO panoptic segmentation data is a rich resource that combines the attributes of the COCO dataset with panoptic segmentation. While it’s primarily used for research and development, ensuring its security is vital to maintain the integrity of countless projects that rely on it.
Common Security Risks in Data Annotation
Navigating the vast landscape of data annotation, especially in specialized areas like coco panoptic segmentation and panoptic video annotation, presents a plethora of security challenges. As the intricacies of computer vision segmentation become more pronounced, understanding and mitigating these risks is imperative to maintain the integrity and confidentiality of the data.
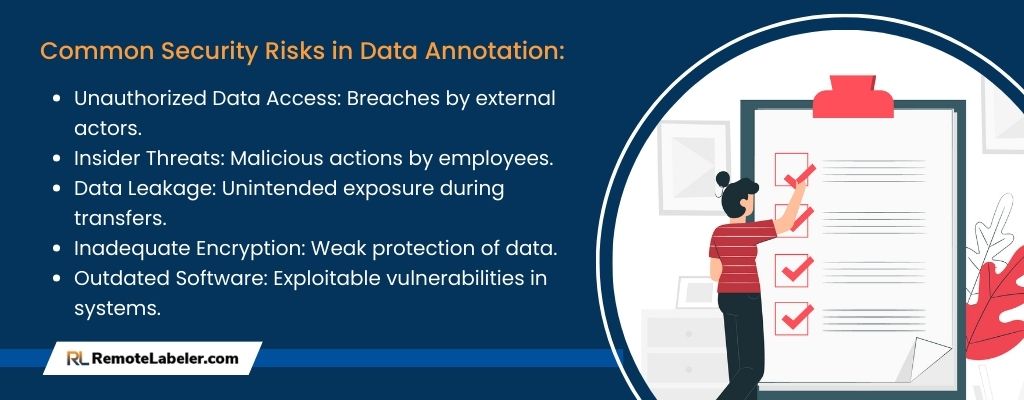
Unauthorized Access
The specter of unauthorized access looms large in data annotation. This risk can manifest due to weak password protocols, absence of multi-factor authentication, or inherent vulnerabilities in the software infrastructure. Breaches of this nature can jeopardize the sanctity of datasets, including those as intricate as coco panoptic segmentation, leading to potential misuse of sensitive information.
Data Tampering
In the realm of data annotation, data tampering signifies the malicious alteration of data. Within the context of panoptic video segmentation and computer vision image segmentation, such tampering can skew results, leading to flawed model training and undermining the very essence of the annotation process.
Data Leakage
The inadvertent exposure or unauthorized sharing of data is termed as data leakage. This is especially critical in areas like medical image segmentation where the inadvertent release of data can not only breach privacy regulations but also expose sensitive patient information, leading to potential legal and ethical ramifications.
Inadequate Data Backup and Recovery
The absence of robust data backup and recovery mechanisms can spell disaster. The risk of losing invaluable annotated data to system malfunctions, human errors, or cyber-attacks is real. Such losses can have cascading effects, leading to financial repercussions and project delays, especially for those relying heavily on these datasets.
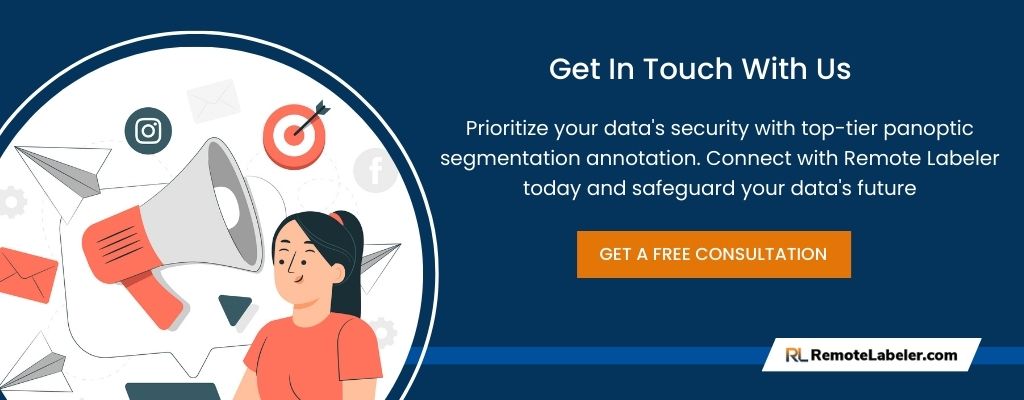
Remote Labeler’s Multi-Layered Security Approach
In response to the multifaceted security challenges inherent in data annotation, Remote Labeler has championed a multi-layered security approach. This strategy encompasses:
- Rigorous Access Controls: Ensuring only authorized personnel have access to specific datasets.
- State-of-the-Art Encryption Methodologies: Encrypting data both at rest and in transit to safeguard against breaches.
- Periodic Security Audits: Regularly reviewing and updating security protocols to address emerging threats.
- Vigilant Monitoring: Proactively monitoring systems for unusual activity to detect and mitigate potential threats early.
By adopting such a comprehensive stance, Remote Labeler ensures that datasets, whether in the realm of computer vision segmentation or medical image segmentation, remain shielded from potential vulnerabilities.
Conclusion
The world of data annotation, especially niches like coco panoptic segmentation and panoptic video annotation, is fraught with security challenges. From unauthorized access and data tampering to data leakage and inadequate backup mechanisms, the risks are manifold. However, with a robust, multi-layered security approach, as exemplified by Remote Labeler, these challenges can be effectively addressed, ensuring the preservation and privacy of data.
Looking for expert data annotation with top-tier security? Choose Remote Labeler for unmatched protection in computer vision segmentation.
- Emerging Trends and Future Outlook: The Data Labeling Industry in 2024-2030 - December 8, 2023
- Landmark Annotation: Key Points - November 6, 2023
- All You Should Know About Bounding Box Annotation - November 5, 2023