The field of artificial intelligence and machine learning is evolving at an unparalleled speed. This growth requires a diverse array of techniques to train models effectively, particularly in the sphere of image recognition and object detection. Among these techniques, Polygon Annotation and Bounding Box Annotation stand as two of the most pivotal methodologies. The choice between these two can significantly impact the precision and accuracy of the training models, thereby affecting the resulting AI application. Therefore, selecting the appropriate annotation method becomes paramount for businesses, particularly when dealing with data collection and labeling.
This article explores the differences between two popular techniques: polygon annotation and bounding box annotation. By understanding their unique characteristics and applications, businesses can make informed decisions when selecting the most suitable approach for their image annotation needs.
Image Annotation and Its Role in Machine Learning
Image annotation is the process of labeling specific objects or regions of interest within an image. It involves the manual or automated delineation of objects, providing contextual information that enables machine learning models to identify and categorize objects accurately. Proper annotation empowers these models to detect objects in real-world scenarios, contributing to advancements in various fields such as autonomous vehicles, facial recognition, medical imaging, and more.
Key roles of image annotation in machine learning:
- Training Object Detection Models: Annotated images serve as training data for object detection models, allowing them to learn patterns and features of objects within images.
- Improving Accuracy: Precise and detailed annotations help enhance the accuracy and reliability of machine learning models by providing accurate ground truth data.
- Enabling Object Localization: Annotation techniques aid in localizing objects within images, facilitating tasks such as object tracking, segmentation, and counting.
- Enhancing Model Generalization: Proper annotation helps models generalize their understanding of objects, allowing them to recognize similar objects in varying environments or viewpoints.
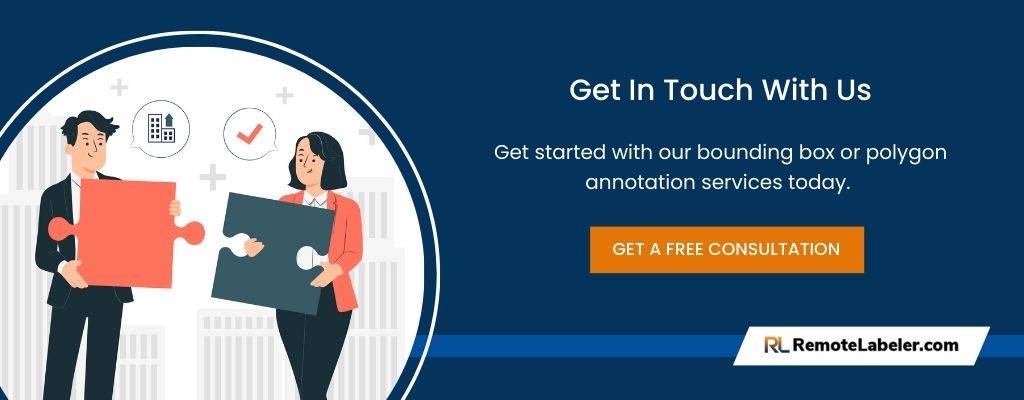
Introduction to Bounding Box Annotation
Bounding box annotation is a widely used method in object annotation, particularly in computer vision tasks. It involves drawing a rectangular box around an object of interest, encompassing the entire extent of the object within the image. The four sides of the box align with the object’s edges, providing a precise representation of its position and size. Here are some key aspects of bounding box annotation:
- Simple Representation: Bounding boxes provide a straightforward and easily interpretable representation of objects, making them widely compatible with machine learning algorithms.
- Efficient Annotation Process: Drawing rectangular boxes around objects is generally faster and less complex compared to other annotation methods, making it a popular choice for large-scale labeling projects.
- Versatility in Object Types: Bounding boxes can be used to annotate a broad range of object categories, including but not limited to vehicles, pedestrians, animals, and various everyday objects.
- Single Object Representation: Bounding boxes are primarily designed to annotate single objects within an image. In scenarios where multiple objects of the same class overlap, bounding boxes may not accurately represent the boundaries of individual objects.
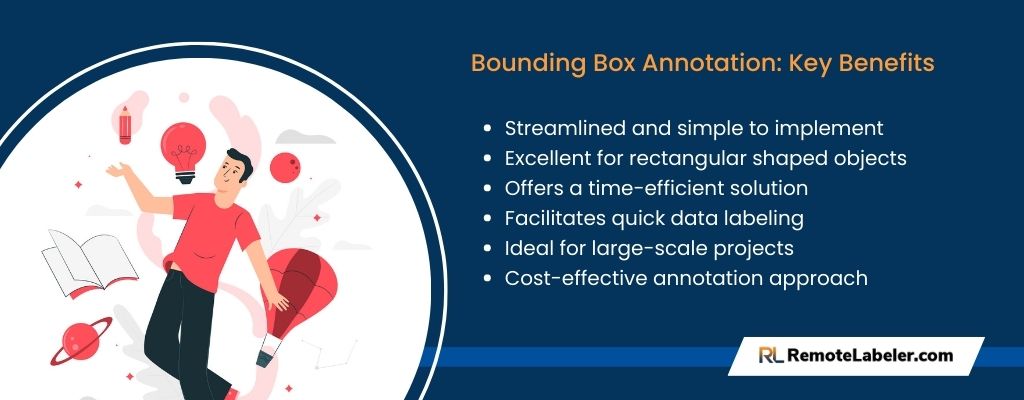
By employing bounding box annotation, businesses can efficiently label large volumes of data and train models to detect and localize objects effectively.
Now that we have covered bounding box annotation, let’s delve into polygon annotation, another widely utilized technique in the field of object annotation.
Polygon Annotation Overview
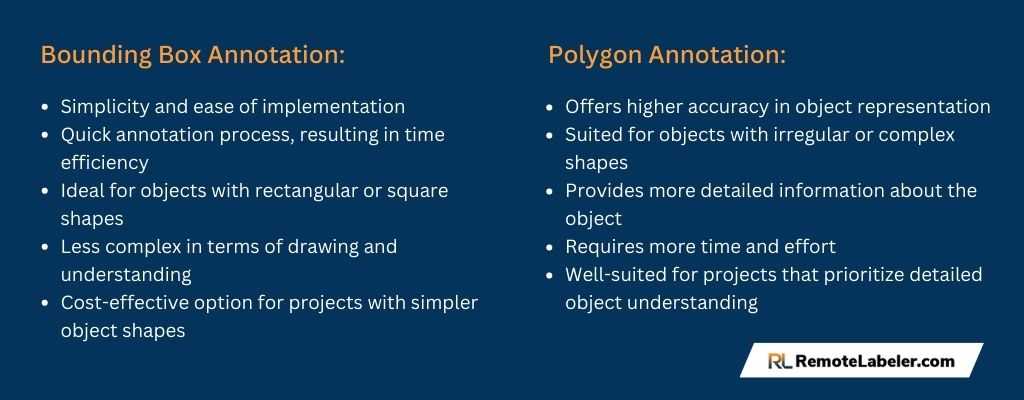
Polygon annotation is a technique used in image annotation where objects of interest are outlined using a series of interconnected vertices. Instead of using rectangular bounding boxes, polygons provide more precise boundaries, especially for irregularly shaped objects or objects with complex contours. Here are some key aspects and benefits of polygon annotation:
- Precise Object Boundaries: Polygon annotation allows for accurate delineation of object boundaries, enabling machine learning models to recognize intricate shapes with higher precision.
- Handling Irregular Objects: Irregularly shaped objects, such as animals, plants, or natural formations, can be better represented using polygon annotations as they provide a flexible and adaptable boundary definition.
- Enhanced Object Segmentation: Polygon annotation assists in segmenting objects from the background, providing finer details for subsequent analysis and understanding of object characteristics.
- Improved Object Recognition: By capturing precise contours, polygon annotations contribute to training models capable of differentiating between objects that might have similar visual characteristics.
- Real-world Applications: Polygon annotation finds applications in diverse fields, including autonomous driving, satellite imagery analysis, medical imaging, robotics, and more, where accurate boundary detection is crucial.
Bounding Box Annotation vs Polygon Annotation: Which One to Choose?
Choosing between bounding boxes and polygon annotation isn’t a straightforward task as it largely depends on the specifics of the project at hand. Here’s a side-by-side comparison of the two annotation methods on different parameters:
Accuracy:
- Bounding Box Annotation: Bounding boxes provide a good approximation of object position and size but may not accurately capture intricate object contours or irregular shapes.
- Polygon Annotation: Polygon annotations offer more precise boundaries, ensuring higher accuracy in capturing complex object shapes and contours.
Efficiency:
- Bounding Box Annotation: Drawing rectangular boxes around objects is relatively quick and straightforward, making it more efficient for large-scale annotation projects.
- Polygon Annotation: Creating polygon annotations can be more time-consuming, especially for objects with intricate shapes or multiple vertices, requiring more detailed annotation.
Complexity:
- Bounding Box Annotation: Bounding boxes are simpler to understand and implement, making them suitable for projects that prioritize simplicity and speed.
- Polygon Annotation: Polygon annotations require more expertise and attention to detail, especially when dealing with complex object shapes or irregular boundaries.
Cost:
- Bounding Box Annotation: Due to its simplicity and efficiency, bounding box annotation tends to be more cost-effective, especially for projects with large datasets.
- Polygon Annotation: The additional complexity and time required for polygon annotation may result in higher costs, particularly for projects that demand precise boundary delineation.
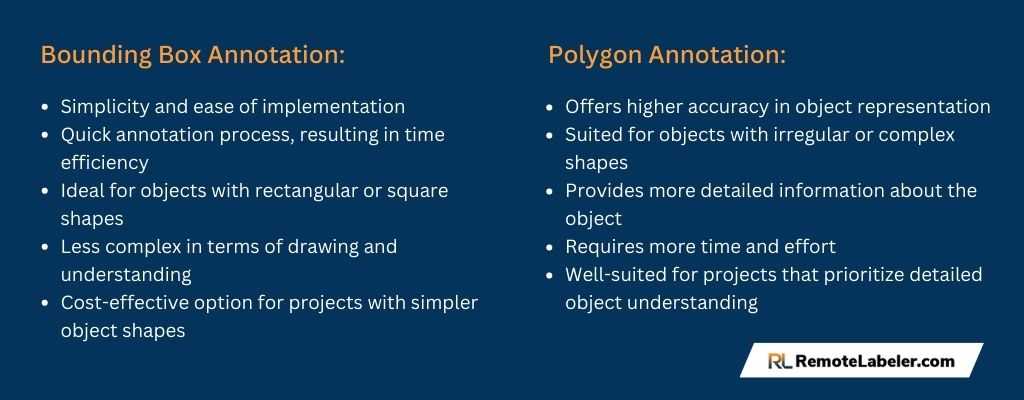
Based on different project requirements, the choice between bounding box annotation and polygon annotation varies. Consider the following guidelines:
- Use bounding box annotation when the objects of interest have regular shapes, and precise contours are not critical.
- Opt for polygon annotation when accurate boundary definition and capturing complex object shapes are paramount, even if it requires more time and resources.
How Remote Labeler Company Can Assist You
In the intricate labyrinth of image annotation, Remote Labeler Company emerges as a beacon, illuminating the pathway for businesses striving to choose the optimal annotation method. We offer a synergistic blend of expertise, technology, and a comprehensive understanding of diverse project needs, paving the way for an annotation approach that fits like a glove.
With an array of services spanning from bounding box annotation to polygon labeling, Remote Labeler Company is uniquely equipped to cater to varied project requirements. Our experts conduct a thorough examination of your project specifications and objectives, subsequently advising on the most suitable annotation method. Whether your project demands the simplicity and speed of bounding boxes or the precision and granularity of polygon annotation, our proficient team is primed to deliver.
For those projects requiring the meticulousness of polygon annotations, we offer top-notch polygon annotation services. Our team of skilled annotators, armed with advanced tools, delivers high-precision labels that significantly boost the accuracy of your machine learning models. Furthermore, if efficiency and cost-effectiveness are your primary concerns, our bounding box annotation services offer swift turnaround times without compromising on quality.
In need of expert guidance in the world of image annotation? Reach out to us today and let Remote Labeler Company empower your AI journey with precision annotation tailored to your unique requirements.
Conclusion
The choice between bounding box and polygon annotation can significantly impact the success of your machine learning project. Each method offers unique advantages and is suited to different project requirements. Bounding boxes provide a simple, quick, and cost-effective solution, ideal for objects with less complex shapes. Conversely, polygon annotations offer a higher degree of precision and accuracy, especially for complex object shapes.
At Remote Labeler Company, we understand the nuances of these methodologies and can help you make the right choice, thereby ensuring that your machine learning models are built on accurately annotated data, driving you towards success. Whether you’re a business venturing into the realm of computer vision or a researcher striving for precision, we’re here to guide you every step of the way.
Choose Remote Labeler Company for your annotation needs, because when it comes to precision, we leave no stone unturned.
- Emerging Trends and Future Outlook: The Data Labeling Industry in 2024-2030 - December 8, 2023
- Landmark Annotation: Key Points - November 6, 2023
- All You Should Know About Bounding Box Annotation - November 5, 2023