In the world where data dictates business strategies, survey sentiment analysis emerges as a beacon of light. It’s an innovative method that dives beneath the surface of customer feedback, enabling businesses to understand not just what their customers are saying, but how they feel. This powerful technique, focusing on data rating & labeling, opens up a goldmine of opportunities by transforming customer sentiments into actionable insights.
Unlike traditional data analysis methodologies, survey sentiment analysis is not limited to categorical feedback. It goes one step ahead to gauge the emotions concealed behind customer responses. Think of it as having the ability to listen to the unsaid, to capture the undertones of satisfaction or dissatisfaction, delight or disappointment, advocacy or aversion. It provides an x-ray vision to see through the veneer of customer communication, tapping into their psyche.
As businesses grapple with ever-evolving market dynamics, staying attuned to customer sentiments can unlock a strategic edge. The potency of survey sentiment analysis lies in its capacity to make sense of massive data quantities, converting them into meaningful insights. It’s a compelling narrative of your customers’ journey, revealing their perceptions, experiences, and attitudes towards your brand, products, or services. It’s a tool designed to empower businesses to navigate the complex maze of customer opinions successfully.
The Importance of Customer Data Analysis
In today’s data-rich business landscape, customer data analysis is much more than a value add—it’s a critical driver of organizational success. It serves as the compass that guides businesses in the right direction, enabling them to stay in sync with their customers’ expectations, preferences, and needs. Businesses that effectively harness customer data can decode the ever-evolving customer behavior, unraveling patterns and trends that often go unnoticed.
While we have already crossed the threshold into the age of the customer, customer data analysis ensures businesses remain in the driver’s seat. With this strategic tool, companies can navigate the intricacies of the customer journey, gaining an in-depth understanding of their customers and making data-backed decisions. Thus, it enables businesses to cultivate lasting relationships with their customers, catalyze growth, and carve out a competitive edge in the marketplace.
Now, let’s dive deeper and explore the inherent importance of customer data analysis:
- Insightful Customer Profiling: Customer data analysis aids in creating accurate profiles, helping in personalized marketing and customer segmentation data analysis.
- Enhanced Decision Making: Rich customer data enables businesses to make informed decisions and forecast trends accurately.
- Improved Customer Service: Through customer service data analysis, companies can refine their services, ensuring customer satisfaction and loyalty.
- Predictive Analysis: This analysis can forecast potential shifts in customer behaviors, helping businesses remain proactive and adaptive.
In essence, customer data analysis is an investment that pays rich dividends. It transforms businesses from being reactive to proactive, allowing them to anticipate customer needs and meet them effectively. Be it optimizing the marketing mix or personalizing customer experiences, customer data analysis makes it all possible. By transforming a plethora of data into actionable insights, it empowers businesses to foster customer loyalty, enhance profitability, and steer clear of the competition. As such, it is an indispensable tool in the arsenal of any business looking to thrive in today’s data-driven world.
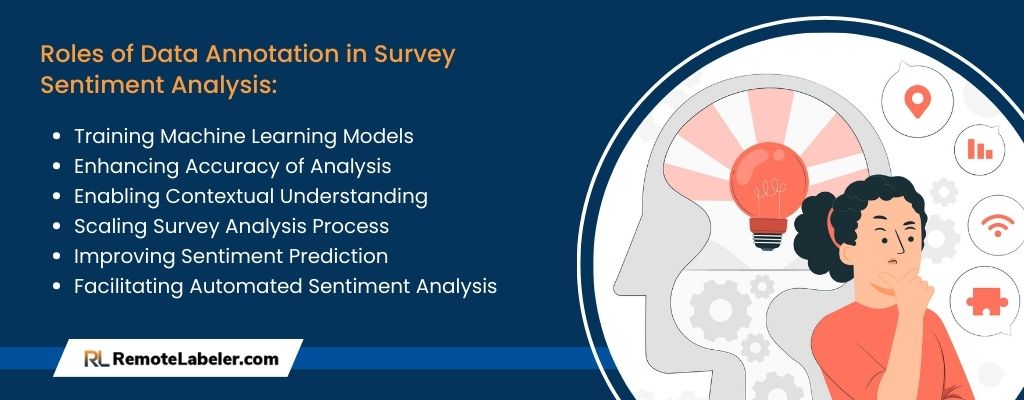
Understanding Survey Sentiment Analysis
Survey sentiment analysis is a complex yet fascinating field that intersects natural language processing, machine learning, and text analysis. In simple terms, it deciphers the emotions hidden within the text data, enabling businesses to understand the sentiment behind customer responses. A sentiment analysis survey is akin to a deep-sea diving expedition that delves into the depths of textual data to unearth valuable customer sentiment pearls.
What sets survey sentiment analysis apart from other data analysis techniques is its ability to derive meaningful insights from qualitative data. The art of interpreting the sentiments in text data requires an algorithmic understanding of language nuances, the interpretation of contextual elements, and the identification of subtle tonal variations. It’s like an elevated form of drug labeling where responses are segregated not just by their literal meaning, but also the sentiment they convey.
Here are a few key aspects involved in survey sentiment analysis:
- Natural Language Processing (NLP): NLP allows computers to understand and interpret human language. In sentiment analysis, it aids in identifying key phrases, interpreting the context, and comprehending sarcasm or irony.
- Machine Learning (ML): ML models are employed to classify text into different sentiment categories such as positive, negative, or neutral.
- Text Analysis: This involves analyzing the text data for tone, emotion, urgency, and other such variables that provide deeper insights into customer sentiment.
In a nutshell, understanding survey sentiment analysis equips businesses with the ability to listen and interpret the voice of their customers. This powerful tool transforms the way businesses interact with their customers, helping them to deliver personalized experiences and foster enduring relationships. By understanding and responding to customer sentiments, businesses can enhance customer satisfaction, boost brand loyalty, and carve out a distinctive competitive edge.
Steps to Conduct Effective Survey Sentiment Analysis
Unlocking the power of survey sentiment analysis is akin to embarking on a meticulously planned expedition, where each step plays a critical role in reaching the desired destination: the extraction of valuable insights. Here, we’ll walk you through the key steps involved in conducting an effective sentiment analysis survey, ensuring that your journey is not only smooth but also rewarding.
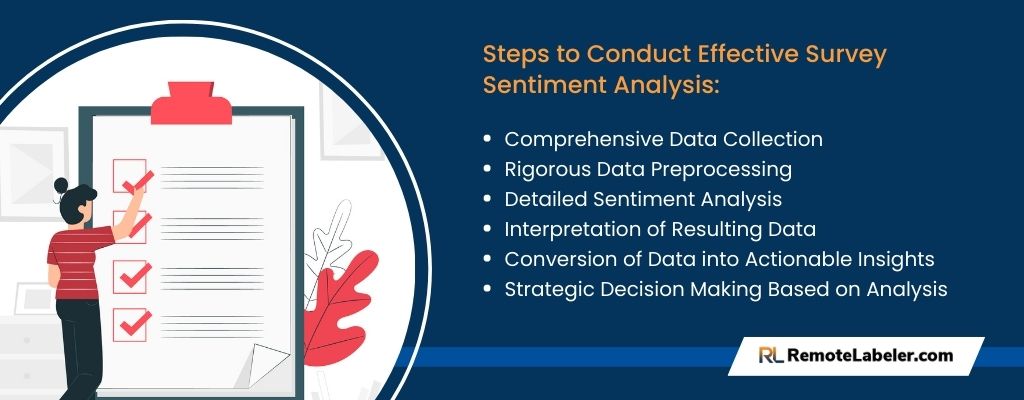
Data Collection
The first step, data collection, is as crucial as laying the foundation stone for a building. It involves gathering qualitative data from various sources, such as surveys, customer reviews, feedback forms, and social media platforms. Remember, the quality of the data collected will determine the accuracy of the sentiment analysis. The broader and more diverse your data set, the more accurate and robust your sentiment analysis results will be.
Preprocessing
Once the data is collected, preprocessing takes center stage. This step involves cleaning and formatting the data to ensure that it’s ready for analysis. It includes tasks like removing stop words (common words like ‘the’, ‘a’, ‘in’ that add noise to the data), stemming (reducing words to their root form), and tokenization (breaking down text into individual words or tokens). Preprocessing is a crucial step as it improves the quality of your data and prepares it for the sentiment analysis process.
Sentiment Analysis
With the preprocessed data in hand, you are now ready for the sentiment analysis. In this phase, the algorithm assigns a positive, negative, or neutral sentiment to each input based on its tone and context. Techniques such as deep learning for sentiment analysis—a survey of various models, can prove beneficial in enhancing the precision of this process. It involves training machine learning models with annotated data to understand and classify sentiments accurately.
Result Interpretation
The final stage of the journey is interpreting the results. This stage is not merely about understanding the results but also about converting them into actionable insights. It involves an in-depth analysis of the sentiment data, identifying patterns, trends, and key takeaways. The interpretation of these results can help businesses make strategic decisions, devise effective marketing strategies, and improve customer service.
In essence, conducting effective survey sentiment analysis is a structured process that involves meticulous planning, data gathering, analysis, and interpretation. Each step is interlinked, with the success of one determining the efficacy of the next. Done right, survey sentiment analysis can open a new dimension in understanding customer behavior and sentiments, driving strategic decision-making and business growth.
The Role of Data Annotation in Survey Sentiment Analysis
Data annotation is the hidden engine that powers survey sentiment analysis. It is the process of labeling data to make it understandable and usable for machine learning algorithms. In the context of sentiment analysis, data annotation involves assigning sentiment values to text data, enabling algorithms to recognize and interpret human emotions. It is an indispensable step in training machine learning models to comprehend the nuances of human language and effectively analyze sentiments.
Think of data annotation as a teacher imparting knowledge to a student. Without annotation, the machine learning model, much like an untaught student, would struggle to understand the data. Data annotation, therefore, ensures that the algorithm can accurately interpret and analyze the text data.
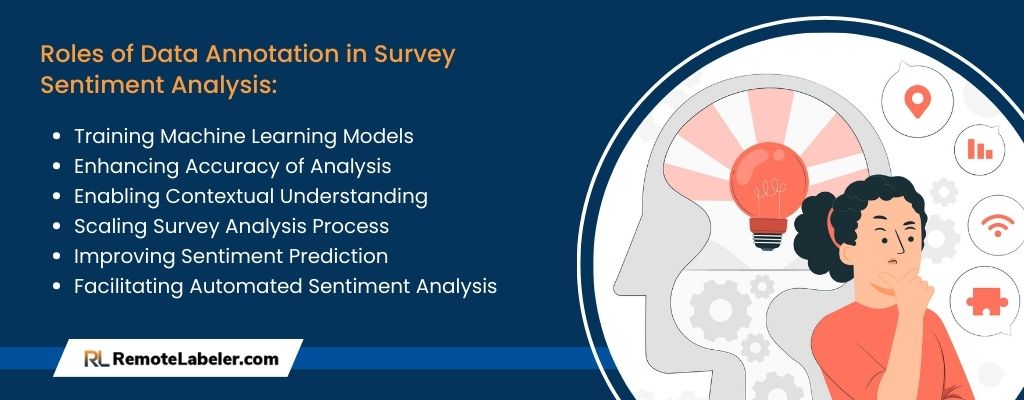
Here are a few critical roles that data annotation plays in survey sentiment analysis:
- Training Machine Learning Models: Data annotation is essential for training machine learning models. It enables the models to learn from the annotated data and gradually improves their ability to classify sentiments accurately.
- Improving Accuracy: By providing labeled data as a reference, data annotation helps in improving the accuracy of sentiment analysis. It acts as a guide, leading the algorithms to make more accurate sentiment predictions.
- Contextual Understanding: Data annotation helps in contextual understanding by labeling the data in a way that reflects its context, tone, and intent. This aids in understanding the true sentiment behind customer responses.
- Scalability: Manual sentiment analysis can be time-consuming and prone to errors. Data annotation facilitates automated sentiment analysis, making it possible to analyze large volumes of data in a short span of time.
Conclusion
From gauging customer satisfaction to fine-tuning marketing strategies, sentiment analysis is a versatile tool that facilitates informed decision-making and strategic planning.
But to leverage its full potential, businesses need to ensure meticulous execution, from data collection to result interpretation. Herein lies the indispensable role of data annotation, a crucial cog in the wheel of sentiment analysis. With precise annotation, machine learning models can decipher the human sentiment intricacies, making sentiment analysis a potent tool in the hands of businesses.
Let Remote Labeler specialists help with it! Get In touch with us for accurate survey sentiment analysis and navigate your business towards success, informed by insights and driven by data.
- Emerging Trends and Future Outlook: The Data Labeling Industry in 2024-2030 - December 8, 2023
- Landmark Annotation: Key Points - November 6, 2023
- All You Should Know About Bounding Box Annotation - November 5, 2023